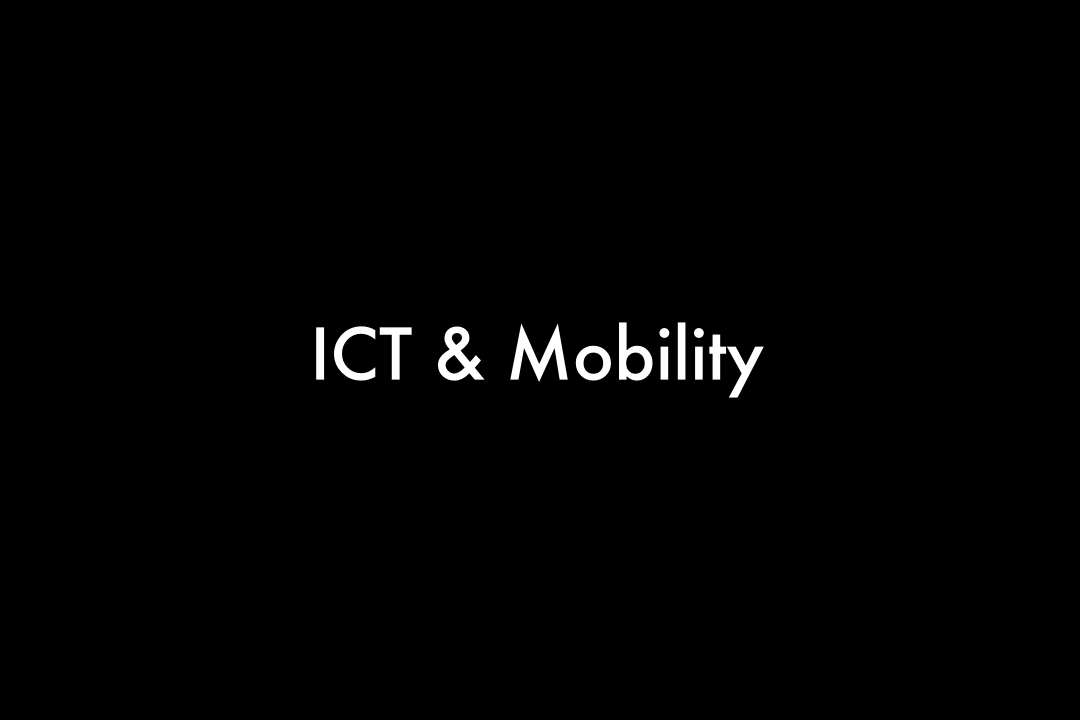
This study focused on evaluating the performance of ensemble learning on handling imbalanced data. Imbalanced data is a special problem in classification task where the class distribution is not uniformed. Resampling (SMOTE and ENN) is employed to improve the classifier performance. Four metrics is applied for performance evaluation i.e. precision, recall, specificity, and F-1 score. Based on the experiments, Bagging has a superior performance compared to baseline classifiers (Naïve Bayes and Log Regression) and other ensemble learnings (Boosting and Random Forest). In addition, the combination of SMOTE and ENN successfully increase the classification performance and avoiding biased to the majority class.